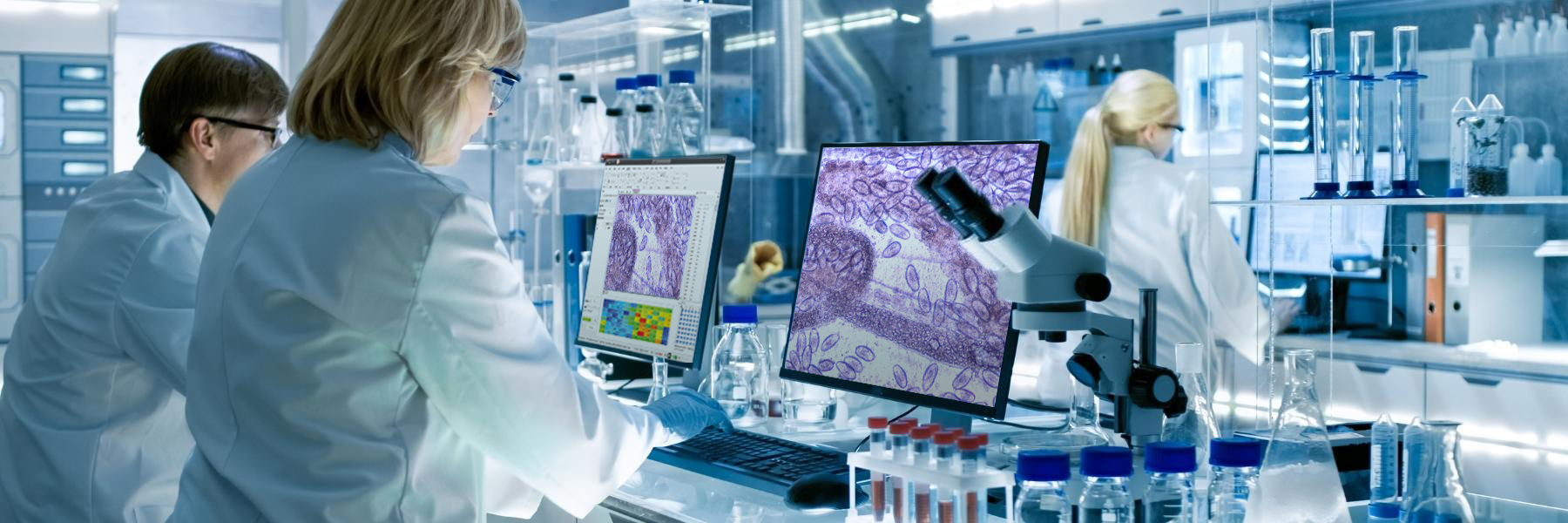
Whether you're working in materials science, pathology, pharma, or basic research, image analysis is likely a central part of your workflow. And while imaging software has come a long way, many of the core image analysis frustrations haven’t changed. Slow manual workflows, irreproducible results, rigid vendor ecosystems, and steep learning curves still haunt many labs.
These issues aren't just annoying; they can delay discoveries, introduce errors, or even undermine the credibility of your work. Below are five of the most common pain-points professionals face in image analysis, with practical strategies and solutions for addressing each—many of which are built into tools like Image-Pro® from Media Cybernetics.
1. Inconsistent Measurements That Undermine Trust
One of the most fundamental goals of image analysis is to produce measurements you can trust, reliably and repeatedly. But in many labs, image quantification still involves subjective manual segmentation, discrepancies across instruments, or variations in how object identification or annotations are selected between users. These inconsistencies are among the most persistent image analysis frustrations and can have serious consequences, especially when they impact publications, regulatory submissions, or quality control metrics.
The NIH Rigor and Reproducibility initiative reinforces just how critical reproducible measurement is for building trust in scientific findings.
How to fix it:
Reproducibility is at the heart of credible scientific research. Regardless of what imaging software you use, it’s crucial to minimize variability by adopting consistent calibration practices, documenting protocols carefully, and reducing subjective steps in image analysis. Use tools that allow you to save settings, standardize workflows, and reduce user-to-user discrepancies.
Image-Pro takes this a step further with global calibration settings, lockable analysis protocols, and AI-powered segmentation tools that reduce reliance on subjective manual input. Its deep learning models adapt to complex features across datasets, helping you maintain consistent measurements and build confidence in your results—even across large teams or datasets.
2. Spending More Time on Manual Tasks Than Actual Analysis
Scientists often find themselves buried in repetitive manual tasks such as outlining objects, removing background noise, managing metadata, or switching between different software. These necessary yet tedious steps can become significant bottlenecks, especially when dealing with extensive sample sets. These time sinks are some of the most frustrating parts of image analysis workflows.
A Nature Methods article emphasized that manual preprocessing and segmentation frequently introduce delays and subjectivity, overshadowing critical downstream analyses.
How to fix it:
If you find yourself doing the same tasks repeatedly (drawing outlines, cleaning images, managing files), it may be time to reevaluate your workflow. Regardless of the platform, look for ways to automate high-friction steps, use batch operations, and explore AI-based segmentation tools that can be trained to handle your specific tasks.
Image-Pro is purpose-built to speed up your work without requiring complex scripts. It offers drag-and-drop automation, reusable analysis protocols, and a growing library of Pre-Trained AI models, allowing you to jump straight into high-value work like interpretation, experimentation, and publishing.
3. No One Around to Help When You’re Stuck
When imaging software becomes mission-critical, users often face delays in getting timely support when issues arise. Online forums can be outdated, help documentation often lacks context, and vendor response times can be slow or impersonal.
This lack of support is one of the more overlooked yet critical image analysis frustrations and can bring projects to a halt, especially when teams don’t have dedicated IT or imaging specialists in-house.
According to the Stack Overflow 2020 Developer Survey, nearly a third of users abandon tools entirely due to poor support and documentation.
How to fix it:
When image analysis becomes mission-critical, having real-time access to knowledgeable support can make or break your momentum. No matter which software you use, prioritize platforms that offer fast, reliable support with domain expertise—not just generic IT help.
Media Cybernetics takes support seriously, offering Success Plans that connect you with experts in microscopy and image analysis, not just troubleshooting agents. Whether you need guidance on segmentation strategy or workflow optimization, you’ll get answers from someone who truly understands your work.
4. Being Locked into a Closed System
Many labs invest in powerful microscopes, cameras, or imaging platforms, only to find that the software they’re expected to use doesn’t work well with external tools. Proprietary file formats, incompatible hardware, or limited import/export options can restrict collaboration and slow innovation. Worse still, they can prevent you from fully leveraging your own data—leading to even more image analysis frustrations.
The FAIR data principles call for open and interoperable data systems that promote reuse and collaboration.
How to fix it:
In today’s collaborative research environment, flexibility is non-negotiable. Seek out software that supports open formats, integrates with your existing hardware, and avoids locking your data behind proprietary barriers. Tools that promote FAIR principles (Findable, Accessible, Interoperable, Reusable,) will serve you better in the long run.
Image-Pro is built with interoperability in mind. It reads over 65 image formats (including proprietary ones), supports a wide range of third-party hardware, and offers seamless data export for analysis in other platforms. This openness keeps your data flowing and your team collaborating, no matter where you work.
5. Software That Requires a Programming Degree
While powerful open-source tools and advanced analysis platforms exist, they often come with a steep learning curve. If you’re not already comfortable coding or configuring plugins, it can take days just to get a simple measurement or export usable data. This creates a barrier for biologists, technicians, and engineers who may not have the time (or interest) to become programmers. This steep learning curve is another of the most frustrating realities of image analysis.
A 2024 article in PLOS Computational Biology argues that reducing the technical complexity of software is key to enabling broader participation in science.
How to fix it:
Complexity shouldn’t be a prerequisite for doing quality science. Whether you’re a technician, a biologist, or a busy PI, choose tools that match your comfort level while still offering room to grow. Look for software with intuitive interfaces, built-in tutorials, and optional advanced features—not a mandatory coding requirement.
Image-Pro is designed with both simplicity and power in mind. Its visual interface allows non-programmers to perform sophisticated analyses without writing a single line of code, while advanced users can tap into scripting tools and APIs for deeper customization. That means your whole team can get up to speed quickly and stay productive longer.
Final Thoughts: Fixing the Foundations of Imaging Work
Many of the frustrations described above aren’t just technical, they’re structural. They reflect a broader disconnect between the evolving needs of scientists and the rigid tools they've been forced to use. Software should support innovation, not slow it down. It should enhance your ability to discover, not force you into someone else’s workflow.
That’s why it’s critical to choose tools that are not only powerful, but human-centered: intuitive, flexible, well-supported, and accurate. Solving these foundational image analysis frustrations doesn’t just make your day smoother—it strengthens the integrity, speed, and impact of your work. In an era when science is increasingly collaborative, data-driven, and fast-moving, that’s a shift worth making.
Media Cybernetics
sales@mediacy.com
Related Links